Share this post
Leap Into the Future: Essential AI Skills for Modern Product Managers
Kevin Weil, Chief Product Officer at OpenAI and Mike Krieger, Chief Product Officer at Anthropic on stage together talk about what AI will mean for product mangers -- and what it's like to be a CPO at an AI company
October 25, 2024

This summary was mostly written by Storytell based on a conversation between Sarah Guo, VC, Kevin Weil, Chief Product Officer, OpenAI, and Mike Krieger, Chief Product Officer, Anthropic .-- you can find the prompt I used here.
You can also join our upcoming webinar on 10/31: Master AI for Product Management: Take Your PM Skills to the Next Level
Here's what Mike and Kevin had to say at Lenny Rachitsky's "Lenny & Friends" product manager summit:
Here are key skills and focus areas Mike and Kevin say PMs should concentrate on as AI advances:
- Technical Acumen in AI:
- Why Important: Understanding AI technologies' basic architecture and working principles is crucial for PMs. This knowledge enables them to evaluate technical trade-offs, collaborate more effectively with data scientists and engineers, and drive product vision.
- What is Changing: The AI landscape evolves rapidly, with capabilities advancing every few months. PMs must now stay informed about these developments to envision new possibilities for product innovation continuously.
- Data Interpretation and Evals:
- Why Important: Modern AI models require rigorous testing and evaluation (evals) to ensure performance and reliability. PMs must develop competencies in designing and interpreting evals.
- What is Changing: Product success in AI is moving towards a more nuanced understanding of model performance beyond standard metrics, necessitating a deeper dive into evaluation frameworks to understand where models excel or fail.
- User-Centric AI Design:
- Why Important: AI systems must be designed with the end user in mind, ensuring that they enhance user experience rather than complicating it.
- What is Changing: AI systems exhibit non-deterministic behavior, and product designs need to accommodate variability in AI responses, focusing on creating seamless user experiences despite these uncertainties.
- Interdisciplinary Collaboration:
- Why Important: With AI bridging multiple domains, working effectively across cross-functional teams is paramount. PMs must mediate between technical and non-technical teams to align product objectives.
- What is Changing: The increased complexity of AI products means integrating diverse expert insights to create impactful AI solutions effectively.
- Strategic Prototyping and Experimentation:
- Why Important: Rapid prototyping using AI can help in quickly validating ideas and iterating product concepts.
- What is Changing: The pace of AI development requires PMs to lead more agile, experimental approaches in product strategy to maintain competitiveness and responsiveness to technological shifts.
- Ethical Considerations and Bias Management:
- Why Important: AI models can inadvertently reinforce biases present in training data. PMs must ensure ethical guidelines are embedded in product development to foster responsible AI usage.
- What is Changing: As AI becomes pervasive, there is growing scrutiny over its impact, urging PMs to prioritize ethics as a core pillar in product strategy.
What it's like being a product manager at an AI company:
Being a product leader at an AI company is both thrilling and demanding, as highlighted by Kevin and Mike from OpenAI and Anthropic during their discussion. Here are some specific insights and examples about the nature of the role:
- Constant Evolution of AI Capabilities: As a product leader, you don't work with a fixed technology base. The capabilities of AI change quickly, sometimes every couple of months. This means that as a product leader, you need to constantly reassess how these developments can alter your product roadmaps. For example, new AI capabilities can be like "magic" discovered inside the company that can unexpectedly transform what your products can do.
- Adapting to Enterprise Needs: Enterprise clients have needs beyond just the technology. For instance, an AI product may require a 60-day advance notice of new launches, which can be challenging to provide. The complexity of aligning AI product capabilities with enterprise client's existing workflows and requirements is a constant balancing act.
- Diverse Stakeholder Interaction: Being a product leader requires engaging not only with customers but also internally with teams like research who inform product capabilities. Product leaders often find themselves partnering closely with researchers to understand and predict emerging model capabilities.
- Handling Uncertainty in Product Capabilities: AI models might perform a task 60% correctly or excel close to 99%. This uncertainty affects product design decisions. For example, GitHub Copilot, even at less than perfect coding capabilities, showed significant value as a product by doing part of the tedious work which developers then refine.
- Importance of Designing with AI's Strengths and Weaknesses: A key insight was about using AI effectively even at 60% capability by involving humans in the loop. This approach helps in building valuable products where AI assists rather than completely replaces human input.
- Emphasis on Evaluation Methods (Evals): Developing good evaluation metrics for AI capabilities becomes critical. Both leaders emphasize the importance of writing good evals and understanding how to assess AI models accurately to measure product success.
- Prototyping with AI: Product leaders and teams benefit from being able to quickly prototype and test products using AI models. The ability to run A/B tests and experiments efficiently helps in refining the product offering.
- Non-Deterministic Nature of AI: Unlike traditional software, AI doesn't always produce the same output for the same input, complicating bug tracking and product testing processes. As a result, product leaders need to establish new feedback mechanisms and guardrails.
- Customer Education: Educating end-users, especially in consumer and enterprise sectors, about how to effectively use AI products is crucial. This includes using the product itself as an educational tool to guide users, like explaining features or navigating interface challenges using chatbots or guides embedded in the product.
- Anticipating and Adapting to Change: The rapid pace of advancements requires a culture of adaptation. Product leaders must foster an environment where their teams and end-users are ready for continual changes in AI capabilities and product offerings.
These aspects make the role of a product leader in an AI company uniquely dynamic, requiring a blend of strategic foresight, technical understanding, and user-centric design thinking.
Gallery
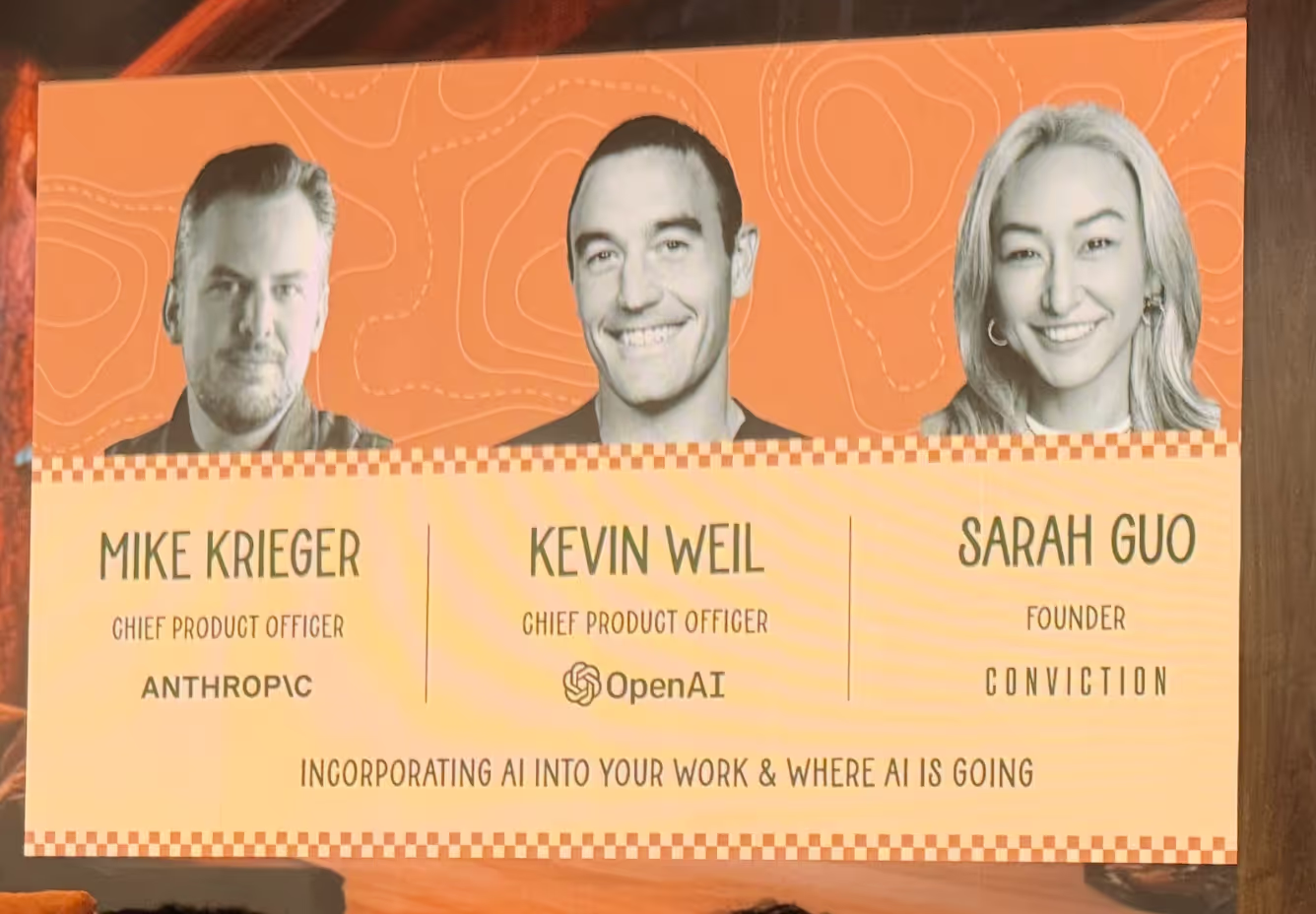

No items found.
Changelogs
Here's what we rolled out this week
No items found.